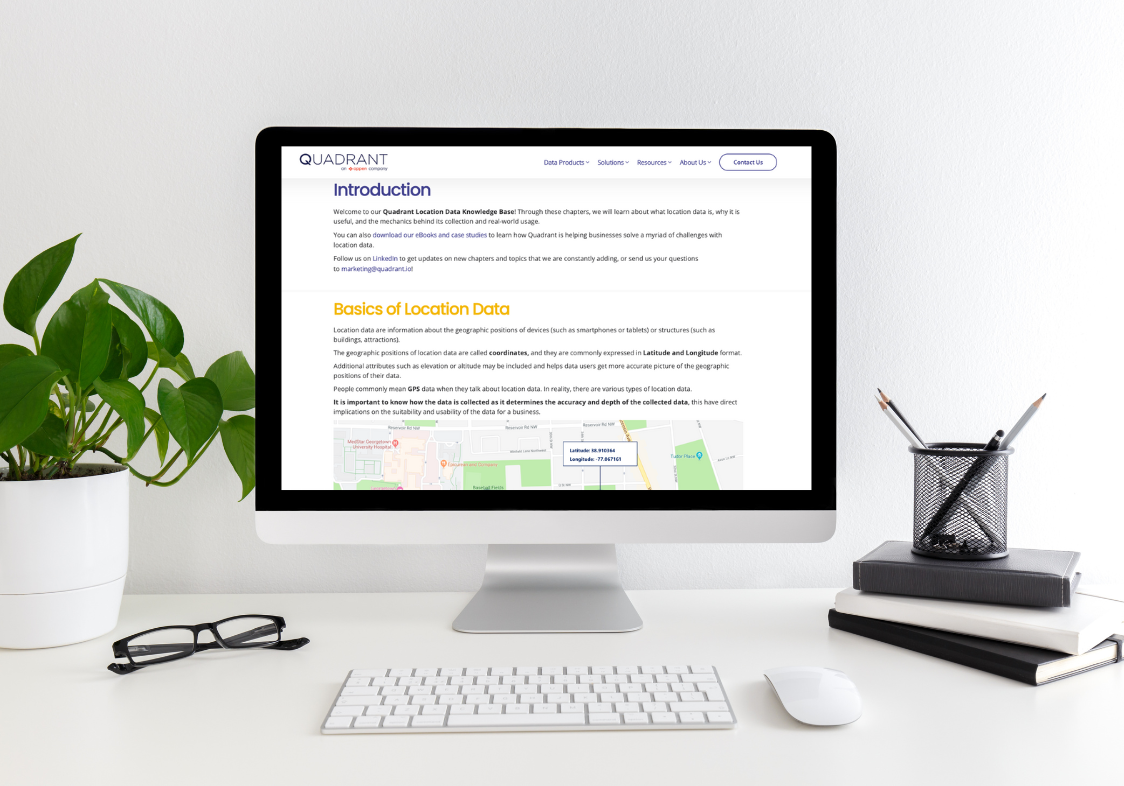
Location data knowledge base
You want to start using location analytics and intelligence to improve your business profitability but do not know where to start? Read on to learn all you need to know about location data and geospatial intelligence.
Introduction
Welcome to our Quadrant Location Data Knowledge Base! Through these chapters, we will learn about what location data is, why it is useful, and the mechanics behind its collection and real-world usage.
You can also download our eBooks and case studies to learn how Quadrant is helping businesses solve a myriad of challenges with location data.
Follow us on LinkedIn to get updates on new chapters and topics that we are constantly adding, or send us your questions to marketing@quadrant.io!
Basics of Location Data
Location data are information about the geographic positions of devices (such as smartphones or tablets) or structures (such as buildings, attractions).
The geographic positions of location data are called coordinates, and they are commonly expressed in Latitude and Longitude format.
Additional attributes such as elevation or altitude may be included and helps data users get more accurate picture of the geographic positions of their data.
People commonly mean GPS data when they talk about location data. In reality, there are various types of location data.
It is important to know how the data is collected as it determines the accuracy and depth of the collected data, this have direct implications on the suitability and usability of the data for a business.
Location Data Sources and Types of Location Data
A GPS provides latitude-longitude coordinates gathered by the hardware on a device which communicates with a satellite such as a car navigating system, a mobile phone or a fitness tracker.
The latitude/longitude coordinates generated by the GPS are considered the standard for location data. Your device receives signals from the satellites and it can calculate where it is by measuring the time it takes for the signal to arrive.
This produces very accurate and precise data under the right conditions. The quality of a GPS signal degrades significantly indoors or in locations that obstruct the view of multiple GPS satellites.
GPS data can be pulled directly from its point of origin - mobile devices - through in app SDKs or via a Server-to-Server (S2S) integration with app publishers.
Data collected from mobile apps have the potential to be very accurate and insightful. User's movement patterns can be observed in aggregates to uncover deeper insights for businesses. However, the biggest challenge with this method is achieving scale.
These apps require a user’s permission to collect location information obtained generally using an opt-in interface when the user first interacts with the application. With newer restrictions on inter-app tracking, some apps only provide location data when they are open or running in the background as chosen by the owner of the device.
Bidstream data is data collected from the ad servers when ads are served on mobile apps and websites. Bidstream data is easy to obtain and scale, but it is often incomplete, inaccurate, or even illegitimate.
Many ads do not collect the location but record the IP address the phone is connected to, This IP often does not reflect the actual location of the device, e.g., a person sitting in a Starbucks but connected to the university campus Wi-F will be recorded as being at the campus. Moreover, due to speed at which ads need to be delivered, very often the cached location of the phone is getting recorded as the GPS does not have enough time to update itself.
Centroids, or a large accumulation of device IDs in central locations, are very common with Bidstream data, as many devices may connect to the same IP address and many apps automatically default to the central location of the country where the ad is served.
Beacons are hardware transmitters that can sense other devices when they come into close proximity.
The location data collected by beacons is very accurate. They can also collect details such as name and birthdays, which can be very valuable to businesses.
Since beacons are hardware, they have to be purchased and installed at locations businesses want to track. Therefore, as with SDK data, it can be challenging to achieve scale through this method.
Wi-Fi enables devices to emit probes to look for access points (routers).
These probes can be measured to calculate the distance between the device and the access point. The precision of Wi-Fi location data is entirely dependent on the Wi-Fi network it is built on.
Wi-Fi networks are great at providing accuracy and precision indoors. Devices can use this infrastructure for more accurate placement when GPS and cell towers are not available, or when these signals are obstructed.
POS data is data that stems from consumer transactions. This data usually contains adjacent information such as purchase items, amount spent, and method of payment, which can provide valuable information.
Because POS data is decentralized, it would be difficult to match multiple data sources through this method. POS data also only captures customers who have made an in-store purchase, and does not capture information on people who entered the store but did not buy anything.
How do I get location data?
There are advantages and disadvantages to each source of location data.
Businesses should consider various factors such as budget, accuracy requirements, and use cases when evaluating the source of their location data.
Businesses should also consider the ways different types of location data can compliment each other.
Most businesses usually purchase location data or location data feeds from data providers. As they do not have the time, resource, and expertise to collect location data.
However, businesses should be aware that the quality of data from each data provider will vary. Data providers that specialise in providing location data tend to have higher quality data, while the more general data vendors might not have the expertise to provide good quality data.
Due to the nature of data, it is near impossible to verify if a provider is selling authentic data. Businesses should assess the credibility of the data provider to avoid purchasing poor quality or even fradulent data.
Quality location data is important as it correlates with the accuracy and reliability of the findings and insights. Bad data can result in false findings, which causes businesses to waste lots of time, effort, and money.
Is it legal to use mobile location data?
Unlike the use of data in the digital world (e.g., user data collected on social media), location data is free of context, i.e., it doesn’t record a person’s identity, demographics, or any other personally identifiable information. Businesses worldwide are using location data for the betterment of services, performing studies to improve lives, and solving numerous other challenges.
However, just like with any such information, consent conditions are applicable to the collection of location data. Data privacy laws like GDPR and CCPA empower users to take ownership of their information and govern how businesses are using it.
Under these consent conditions, data collectors must gain the consent of customers to use, store, manage, and share their data, while allowing them to modify or opt-out of their earlier preferences at any point in time.
Download our eBook to learn what is consent management, why it is important and how you can establish compliance with the stringent consent requirements mandated by today's data privacy laws.
Representing Location Data
Latitude / Longitude
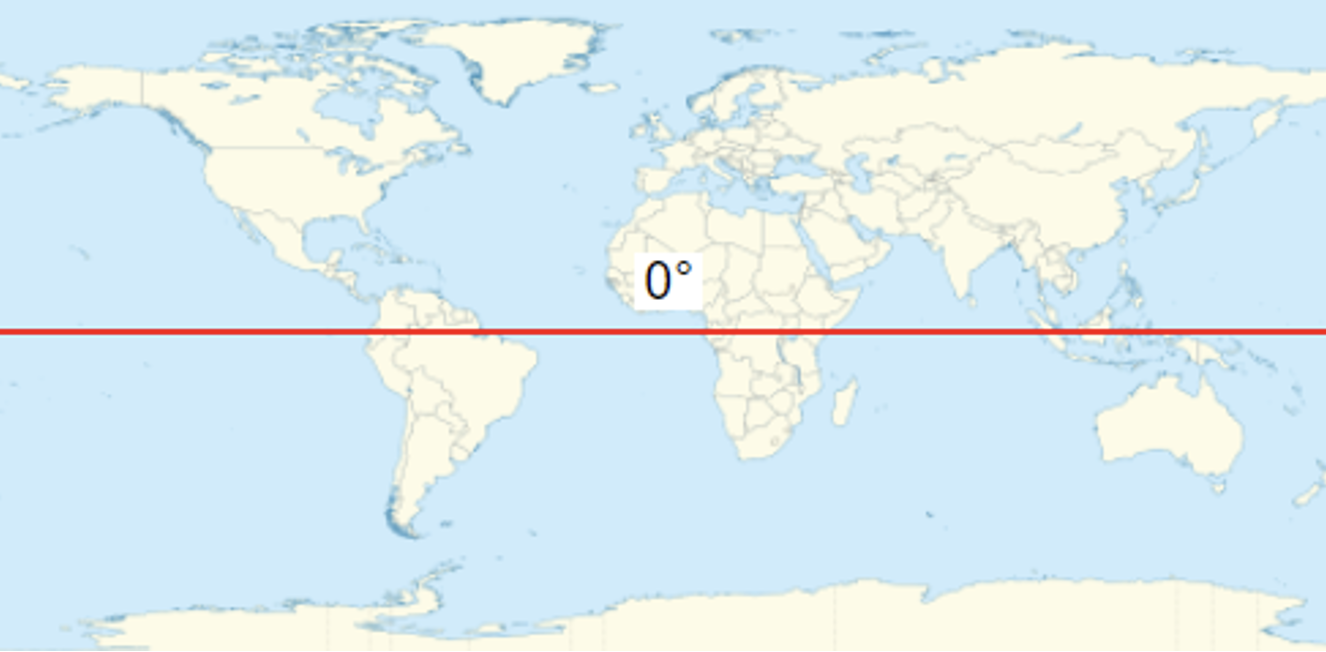
The "latitude" of a point on Earth's surface is the angle between the equatorial plane and the straight line that passes through that point and through (or close to) the center of the Earth. The 0° parallel of latitude is designated the Equator, the fundamental plane of all geographic coordinate systems.
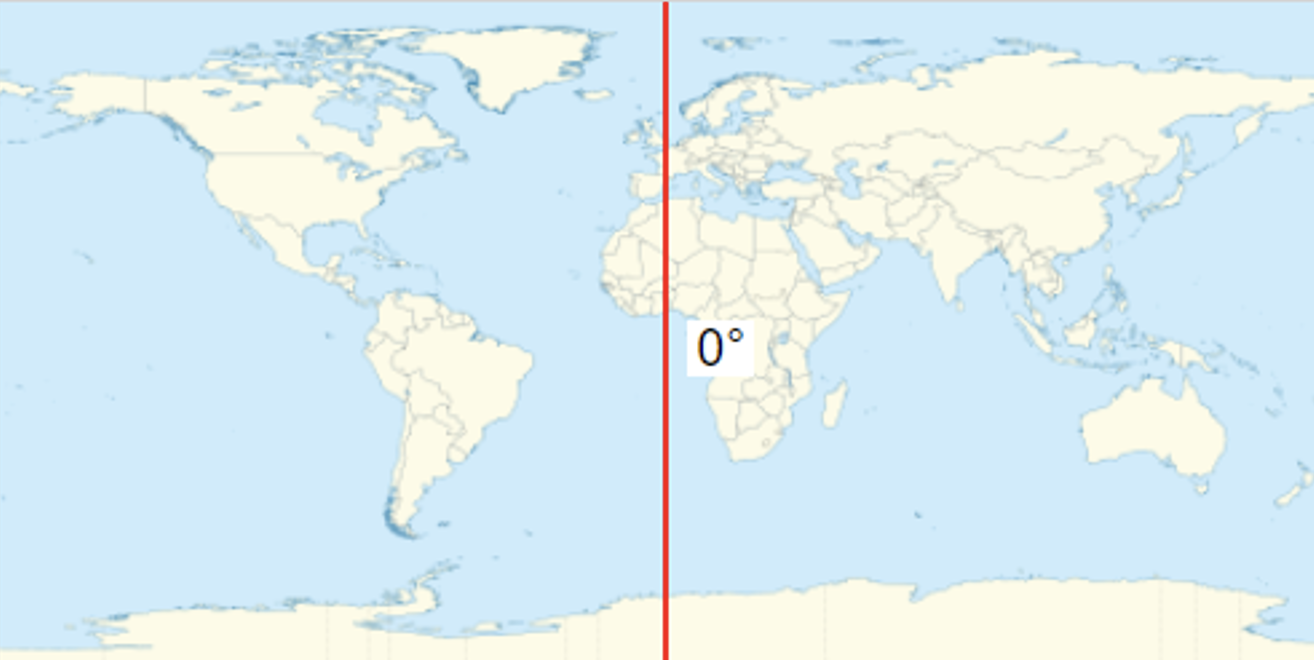
The "longitude" of a point on Earth's surface is the angle east or west of a reference meridian to another meridian that passes through that point. Fun fact, you can actually step on the meridian if you ever visit British Royal Observatory in Greenwich, in southeast London (highly recommend it as the view of the city is amazing from there).
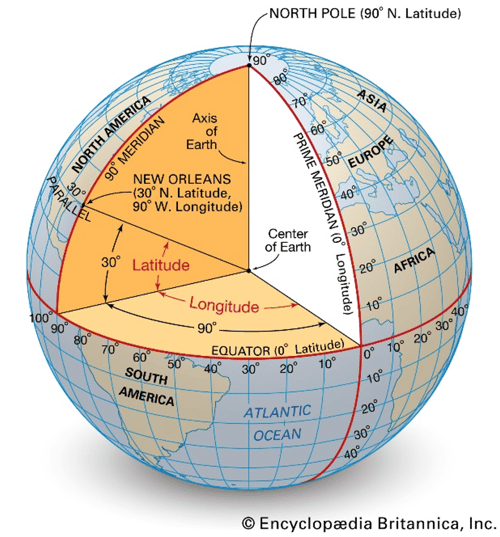
The combination of these two components specifies the position of any location on the surface of Earth. Lat/long data points can be expressed in decimal degrees (DD). The other convention for expressing lat/long is in degrees, minutes, seconds (DMS). For example, below is the same point expressed in DD and DMS (you can find many converters online):
DD: 47.21746, -1.5476425
DMS: 47° 13’ 2.856”, 1° 32’ 51.5106”
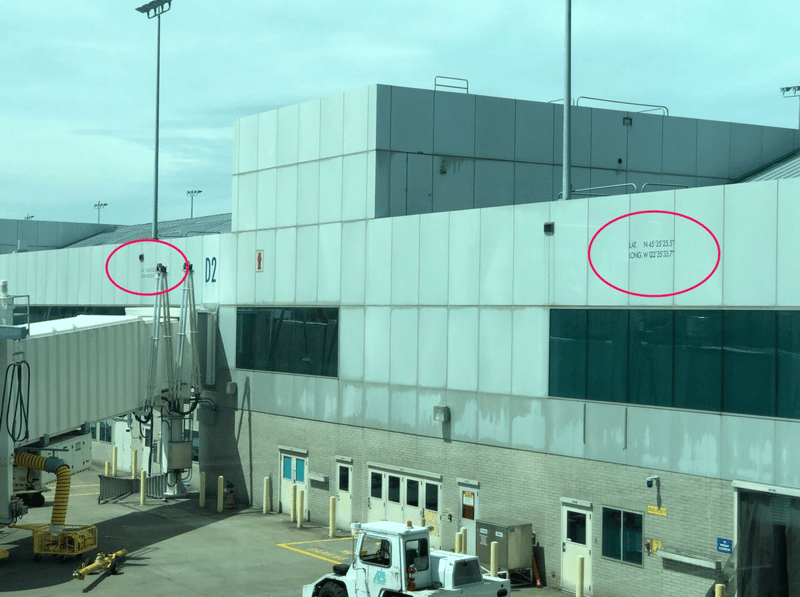
You can see these DMS coordinates at airports, where the gates are marked in degrees, minutes and seconds
Another important thing to understand about decimal degrees is that they carry a level of precision. The number of decimal places required for a particular precision at the equator is:
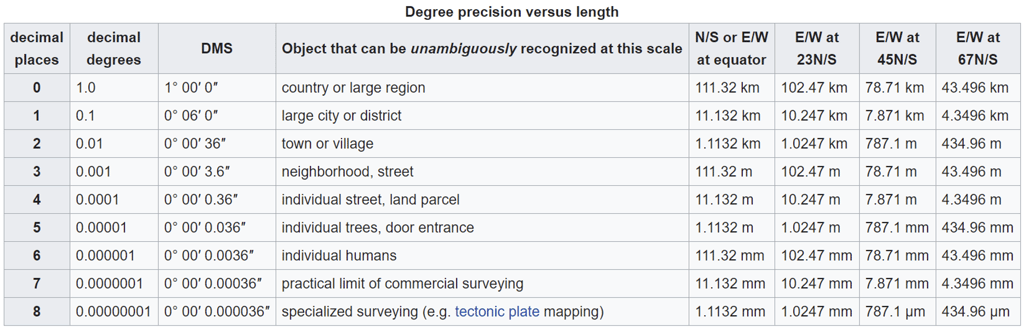
A value in decimal degrees to a precision of 4 decimal places is precise to 11.132 meters at the equator. A value in decimal degrees to 5 decimal places is precise to 1.1132 meter at the Equator.
Geohash
Invented by Gustavo Niemeyer, Geohash is a geocoding system that allows the expression of a location anywhere in the world using an alphanumeric string. Geohash is a unique string derived by encoding and reducing the two-dimensional geographic coordinates (latitude and longitude) into a string of digits and letters. A Geohash can be as vague or accurate as needed depending on the length of the string.
Geohashes use Base-32 alphabet encoding i.e., uses all digits 0-9 and almost all lower-case letters except "a", "i", "l" and "o". It is a convenient way to express a location anywhere in the world. Geohashes basically divide the world into a grid with 32 cells. Each cell will also contain 32 cells, and each one of these will contain 32 cells (and so on repeatedly).
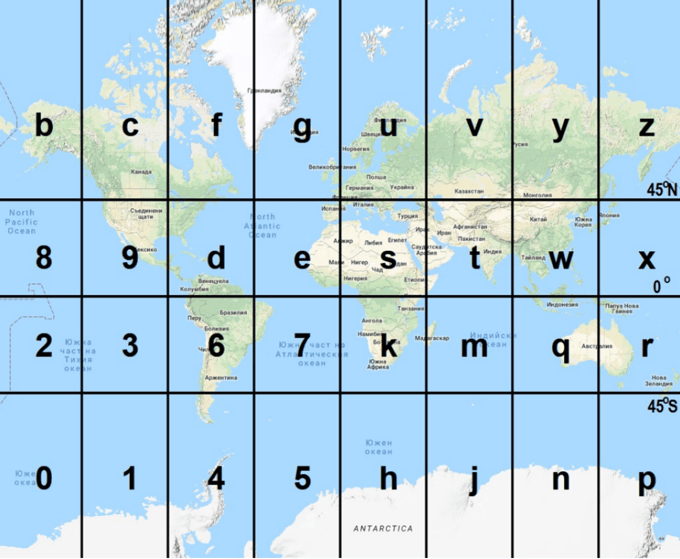
Adding characters to the geohash sub-divides a cell, effectively zooming in to a more detailed area. This is referred to as geohash precision. Geohash Precision is a number between 1 and 12 that specifies the precision (i.e., number of characters) of the geohash. Each additional character of the geohash adds precision to your location.
At Quadrant, we usually provide 12-precision geohash for all the events.
The cell sizes of geohashes of different lengths are as follows; note that the cell width reduces moving away from the equator (to 0 at the poles):
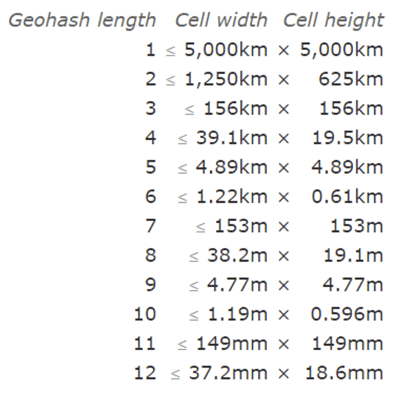
Visually:
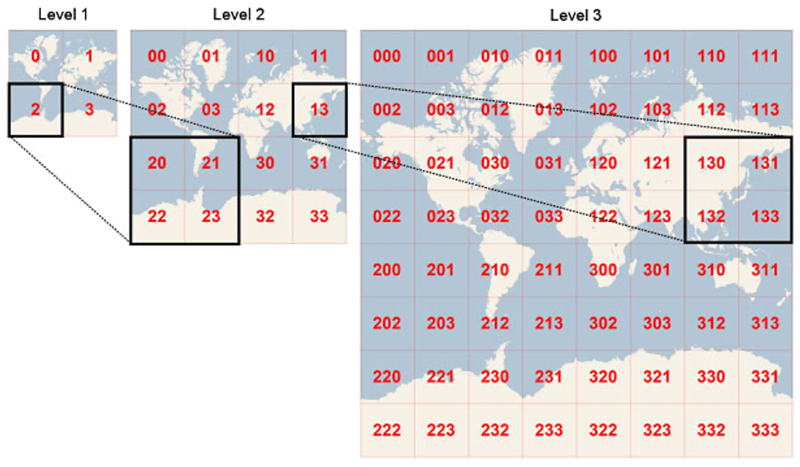
Geohashes have a certain property that makes them suitable for geospatial queries like localized search (points with similar geohashes that are near each other with the same geohash prefixes).
For example, if you want to list the number of persons who were seen in and around the Empire State Building, you can first determine the geohashes you want to cover and then run a simple query:
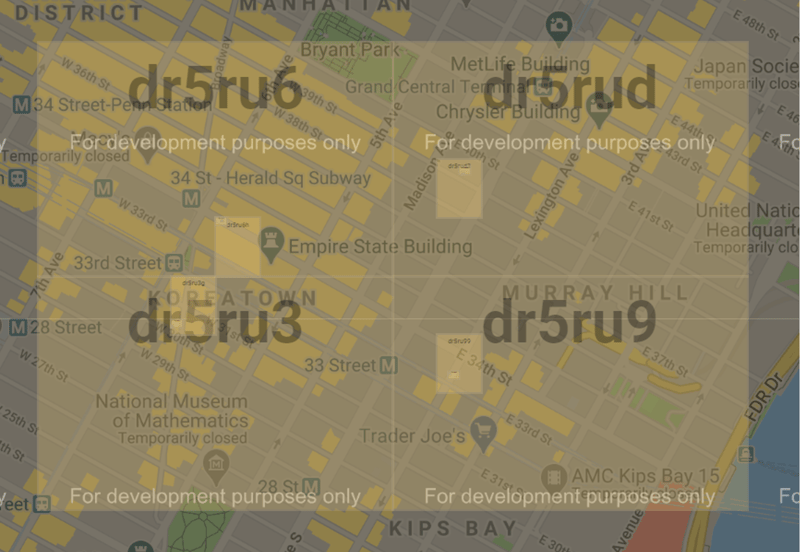
SELECT * FROM table_name WHERE geohash like 'dr5ru6%' or geohash like 'dr5ru3%' or geohash like 'dr5rud%' or geohash like 'dr5ru9%';
Doing this improves processing times and costs, as it allows you to quick sort through large amounts of data and work on more precise subsets of data. In fact, most data scientists use geohash to quickly sort through large location data sets, and then build specific queries (such as polygons) around the specific point/area of interest. In doing so, you can reduce your costs and increase your speed of processing, while maintaining accuracy and precision.
Types of geo-indexing systems
Geodata is information about geographic locations that is stored in a format that can be used with a geographic information system (GIS). For example, at Quadrant, our geo data is stored in three different formats which can be used for geospatial analysis: Country Codes, Latitude & Longitude coordinates, and Geohashes.
Country Codes
Usually the ISO2 2-digit alpha country code represents the locale of the devices i.e. the devices registered to users from the stipulated countries. At Quadrant, in addition to the country code, we also derive another attribute called ‘country’, where the country represents the events / devices that are seen within the geographical boundaries of stipulated countries. For example, if you want to get the total number of events seen within Singapore by using its country code, you can run a simple query: SELECT count(*) FROM table_name WHERE country = ‘SG’;
Lat/long coordinates
Coordinates can be used to identify where an event was recorded. We can use the coordinates to either list devices from a single location: SELECT * FROM table_name WHERE latitude = ‘41.9022’ and longitude = ‘-76.37695’
Or we can use a bounding box, which is an area defined by two longitudes and two latitudes, to get information from a certain area or a country.
Bounding box for Australia:
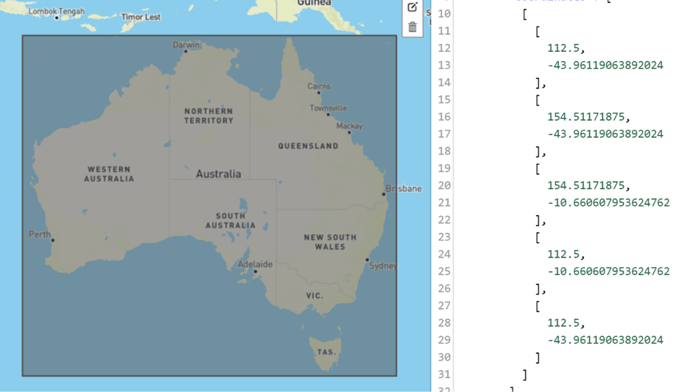
To get the total number of events seen within Australia by using a bounding box, you can run a simple query:
SELECT count(*) FROM table_name WHERE (latitude BETWEEN -43.96119063892024 and -10.660607953624762 and longitude BETWEEN 112.5 and 154.51171875);
Geofencing
A geo-fence is a virtual perimeter for a real-world geographic area. They could be a radius around a single point, or a predefined set of boundary. Once a geo-fenced boundary is defined, the opportunities what businesses can do is limited by only their creativity.
One common use of geo-fencing is for businesses to set up geo-fences around their competitors. And push marketing promotions to customers that enters the zone. This is sometimes referred to as geo-conquest. Businesses could also provide Location Based Services within geo-fenced region.
Geofencing is ideal for catchment area analysis; a catchment is an area from which businesses expects to draw their customers from. Catchment areas can help businesses identify where to run their next marketing campaign or set up their next store.
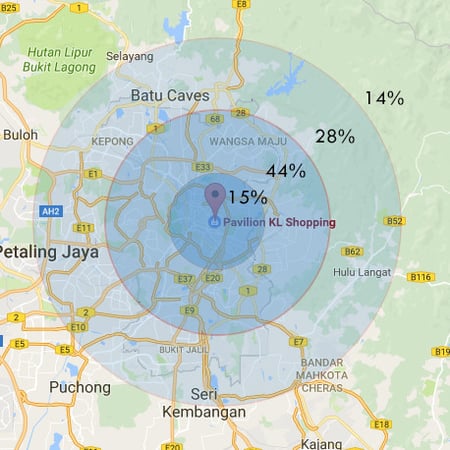
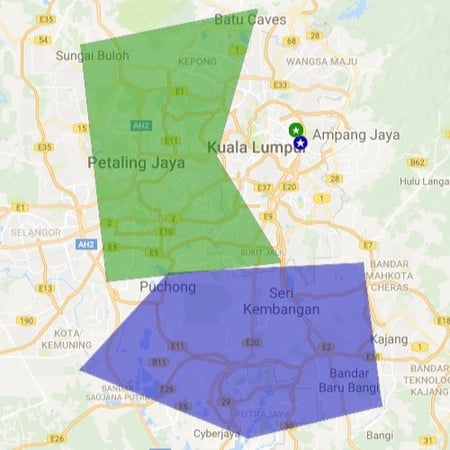
Location Data Attributes and Data Fields
Location data generally have some attributes or data fields in common such as latitude, longitude, and horizontal accuracy. Other data fields tend to be dependent on the source of the data.
Below is a non-exhaustive list of attributes found in location data:
Latitude and longitude shows the position of a device or structure. They are commonly accompanied by horizontal accuracy, which tells users the degree of error in a particular data point.
Altitude or elevation pinpoints the height above a reference point, usually sea level.
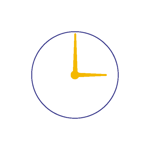
Timestamps are typically used for logging events alone or in a sequence.
In the case of location data, they provide context to the movement of a particular device.
Location data feeds commonly record timestamps in Unix time, otherwise known as Unix Epoch time, or Epoch for short.
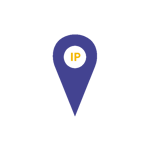
Internet Protocol Address, or commonly known as IP address, is a numerical label assigned to each device connected to a computer network.
IP addresses can be used for location, however, accuracy can be problematic. One common occurrence when looking up an IP address's location is being directed to the network provider's location.
Depending on the business uses, IP address may provide a good rough measure of geographic location.
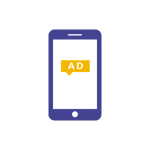
The Mobile Ad ID or Device ID is a unique 36-character identifier of smartphones. Mobile Ad IDs helps to identify, track and differentiate between mobile devices.
The Mobile Ad ID/Device ID is widely used across the marketing ecosystem, especially for targeting devices with ads through demand-side platforms (DSPs) and supply-side platforms (SSPs) in the app advertising supply chain.
For iOS devices the unique device ID is called Identifier For Advertising (IDFA). Apple customers can receive their mobile device ID numbers through iTunes or the Apple App Store. Before the rollout of iOS 6, this device ID was called a Unique Device Identifier (UDID) in the Apple ecosystem.
For Android devices the device ID is called Google Advertising ID (GAID). These mobile device IDs are randomly generated during the first activation of a mobile device and remain constant for the lifetime of the device unless reset by the user. Typically the IDs belonging to iOS devices will be in upper-case and those belonging to Android devices will be in lower-case.
As they are a unique identifier, they can be used to calculate aggregated metrics such as Daily Active Users (DAU), Monthly Active Users (MAU), etc. To learn more about the different queries you can run using the device ID please visit our Resources library.
Horizontal Accuracy
GPS satellites broadcast their signals in space with a certain accuracy. However, this accuracy is not directly controllable by the app developer, at least not through any software mechanism. The accuracy received depends on additional factors such as satellite geometry, signal blockage, atmospheric conditions, and receiver design features/quality.
Horizontal Accuracy is a radius around a 2D point, implying that the true location is somewhere within the circle formed by the given point as a center and the accuracy as a radius. As shown in the example below, the exact location of the device will be anywhere within the orange shaded circle:
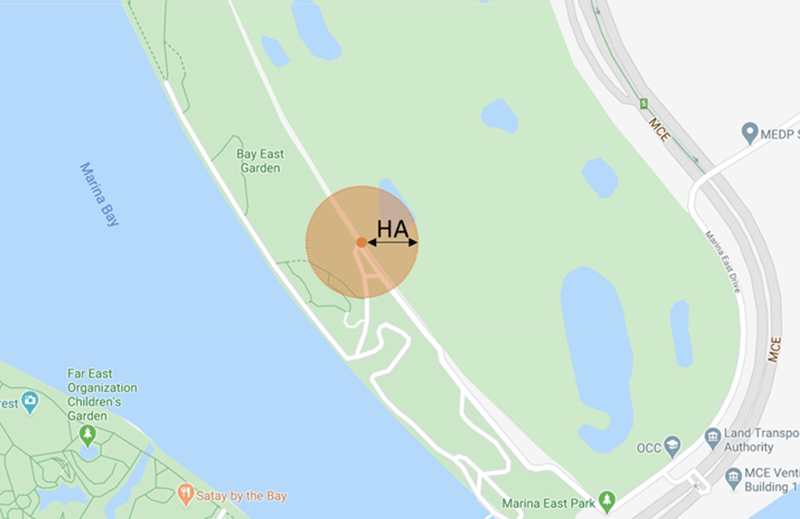
Different use cases require different levels of accuracy readings. For example, a relatively weak level of horizontal accuracy would be acceptable in city- or country-level analyses, as opposed to a more precise segmentation of users that visited stores within a retail park.
It is important to understand the difference between the horizontal accuracy of a point and the precision of a point, which discussed in a previous post, is represented by the number of decimal points in the latitude and longitude coordinates. Simply put, the precision of a point is a measure of how exact the pinpoint location of a data point is on a map. The horizontal accuracy is a measure of how close the data point is to the actual (ground truth) point.
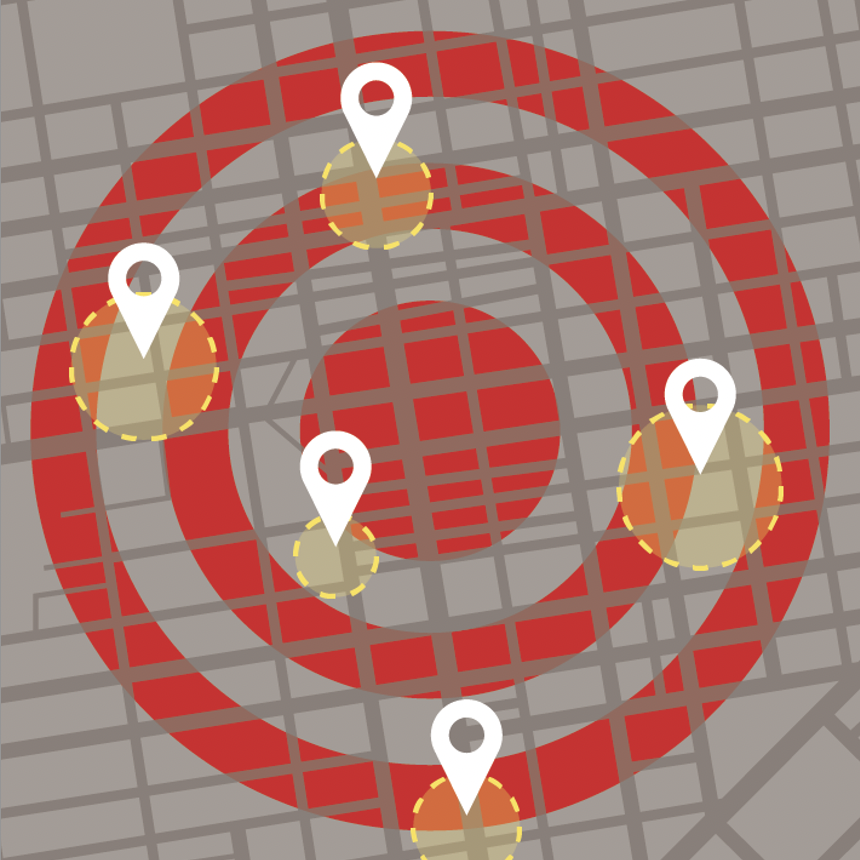
Low accuracy/Low precision
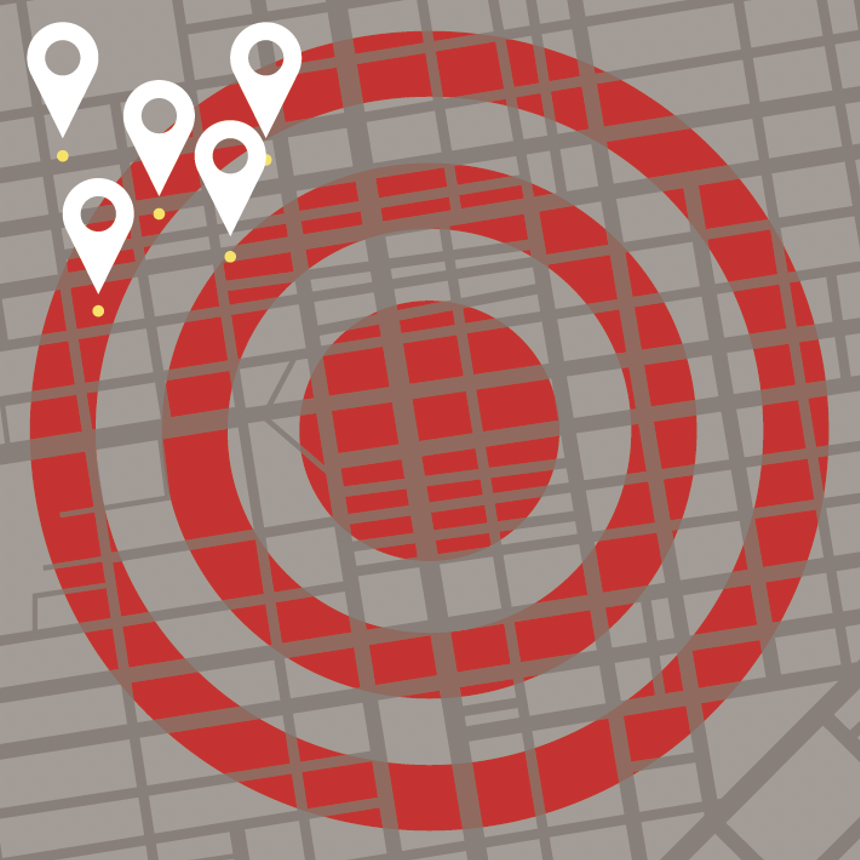
Low accuracy/High precision
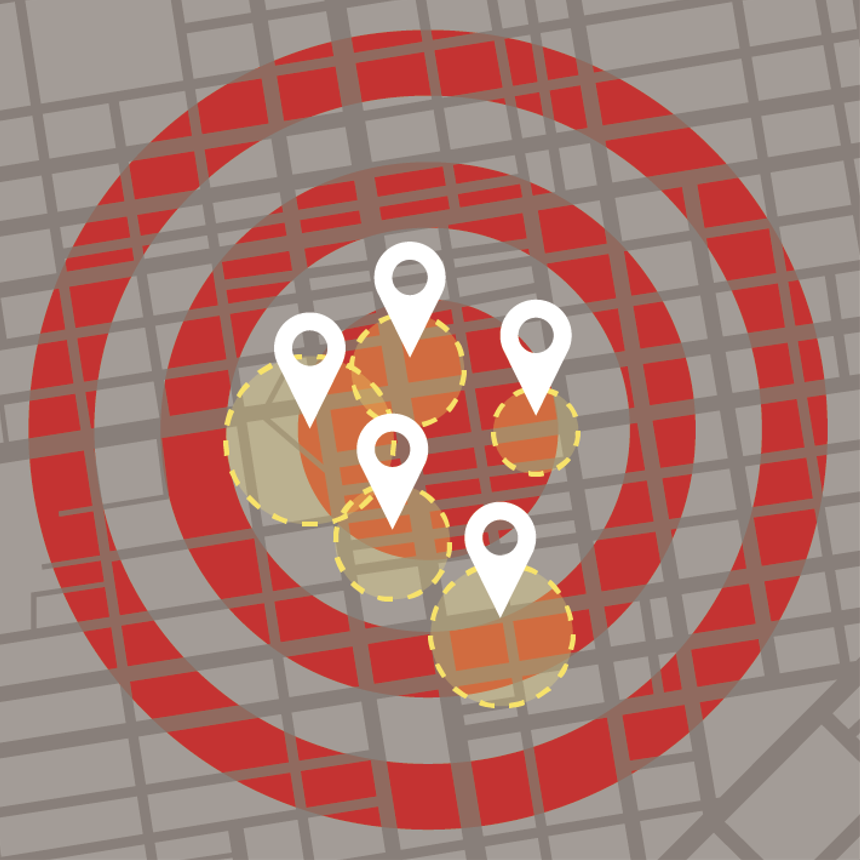
High accuracy/Low precision
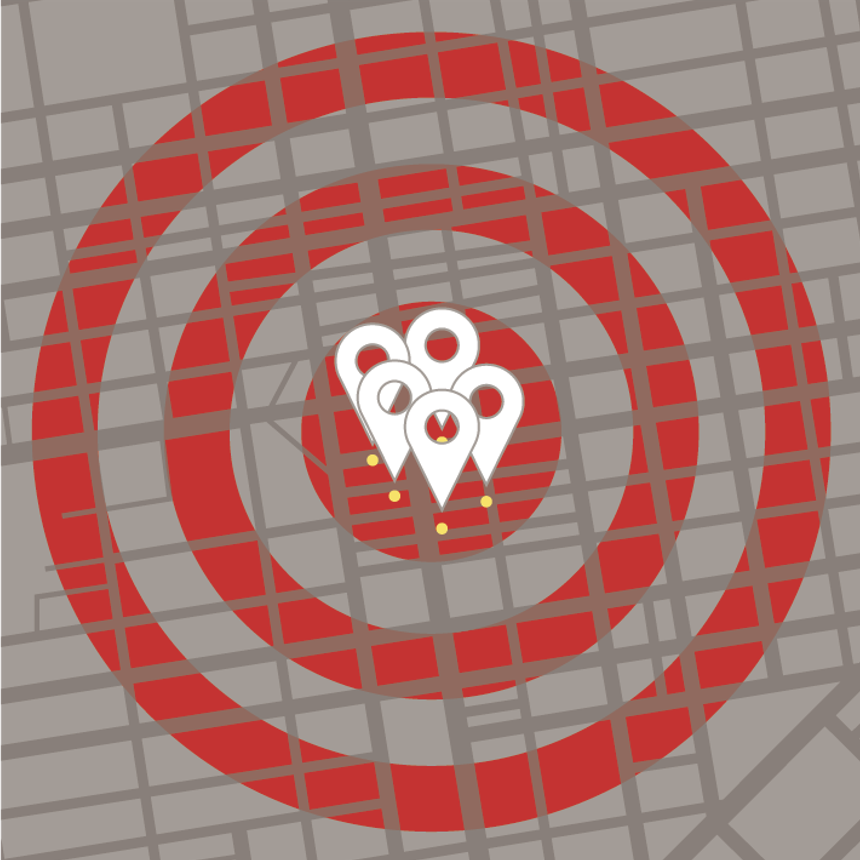
High accuracy/High precision
In summary, if you were to conduct an experiment where you measure the location of a device over time, these two scenarios would describe the difference:
Scenario 1:
High precision means you have low standard deviation from the mean of the distribution (seen on the second and fourth pictures).
For example, the points are measured a small distance apart from each other (all around the Empire State Building, 34th St). The true location of the device may be relatively far away from these points (Chrysler Building, 405 Lexington Ave). However, there is still high precision here because the points are all measured close to each other but low accuracy because they are far off from the true position of the device.
Scenario 2:
High accuracy means that the points you collected are closer to the true location of the device (seen on the third and fourth pictures).
For example, the points are measured close to the true position of the device (Chrysler Building, 405 Lexington Ave), but are not necessarily close to each other (some points recorded at Grand Central Terminal and others at The Westin New York Grand Central, 212 E 42nd St). The precision of these points would be lower compared to the first scenario, but the accuracy would be higher because The Westin and Grand Central Terminal lie closer to the Chrysler building which is the true location of the device.
Still have any questions?
Can’t find the answer you’re looking for? Please contact to our team.
Subscribe to our newsletter
Join our community of 60,000+ active subscribers and stay ahead of the game! Our monthly newsletter provides exclusive insights into the geospatial world, featuring the latest trends, case studies, and cutting-edge innovations.